Gatagoto
Android & iOS apps | From Cyclists For Cyclists
Full-stack Platform | Launched Nov 2019
Berlin is big on biking. More than 80% of Germans cycle, and Berlin has one of the highest rates of bicycle commuting in the world.
Despite the popularity of cycling, it’s clear that things could be a lot better. Although Berlin is more cycle-friendly in terms of cycle lanes and infrastructure in comparison with many other cities, you’ll regularly hear complaints of cycle lanes in bad condition, blocked by fallen tree branches or rendered unsafe by potholes. I know this from talking to regular cyclists and from being a keen cyclist myself.
Cycle safety is a real problem: Between 2017 and 2018, cycling deaths in Berlin increased by 10%, the number of people seriously injured increased by more than 18%, and the number of accidents increased by more than 12%. The government, on a city and national level, have made supporting cycling a priority. But rather than wait for things to improve, we saw an opportunity to do something about the situation ourselves.
Earlier this year, DV in Berlin undertook our first ever internal hackathon. We’re used to building ventures in collaboration with corporate partners and we’ve also hosted hackathons where we work with the local community to innovate towards a specific theme. This hackathon was different: We wanted to see what we could come up with on our own, identifying a problem and putting together a solution. We called it Hack-Our-Future, and projects were aimed at areas for future innovation within DV.
This is where Gatagoto began. Taking cycling infrastructure as our starting point, we honed in on a major problem with the Berlin cycling experience: Badly serviced cycle lanes. Although Berlin has many cycle lanes, often they’re blocked by obstacles on the road, dangerous potholes, incorrectly parked vehicles, or cross dangerous intersections.
We thought about how we could solve this problem and came up with the solution of a software product that would crowdsource issues, report them, and warn other cyclists. After a few ideation sessions, we built an app we called Unter, finishing a basic version within the hackathon.
Unter requires cyclists to take a picture of the issue, add a description of the problem, and send the complaint. It uses Machine Learning Computer Vision capabilities to recognize the problem and categorize it to make reporting easier for the user, who can, if they need to, change the category the algorithm assigns. The complaints are compiled in our dashboard, which collates all issues and, once an issue has been confirmed by other users, we send it to the relevant public authority with the correct tagging, enabling them to easily process issues and prioritize them. All users are able to see the reports collected on a map of the city so they can avoid them if they choose.
The positive reception of Unter at the hackathon was encouraging, and led us to think about whether we could craft our basic ideation version into a full-scale, in-market MVP. We ended up deciding to build out the product as our first DV-led venture, built without a corporate partner.
We called the full app Gatagoto, after the Japanese word for the sound that bicycles make when ridden on an uneven surface that makes it shake.
We built Gatagoto with a full team of developers and designers (listed below), using it as an opportunity to showcase DV’s capabilities when we work alone. The project embodies the DV principle of productive disruption, taking the official online reporting solution of the city authority and challenging it. Gatagoto puts the user first through good design and community contribution.
In the longer term, Gatagoto may support routing, haptic feedback and voice control. We hope it’s useful for everyone who cycles in Berlin, and we’re excited for what it will offer in the future.
I want to thank everyone who made Gatagoto possible.
The Gatagoto team
Core Team:
Tevfik Aloglu,
Maria-Ines Chevallier,
Yue Chi,
Constantine Karlis,
Taichi Kozaki,
Koos Looijesteijn,
Manish Jain,
Kripa Shetty,
Nico Sorger,
Martin Stadler
Support from:
Timothy Clifford
Alexander Grosse
Hainan Hu
Martin Liesenberg
Melissa Sayiner
Dirk Sievert
Tom Stuart
Marcus More-Thiesen
Moiz Zuberi
Featured in:
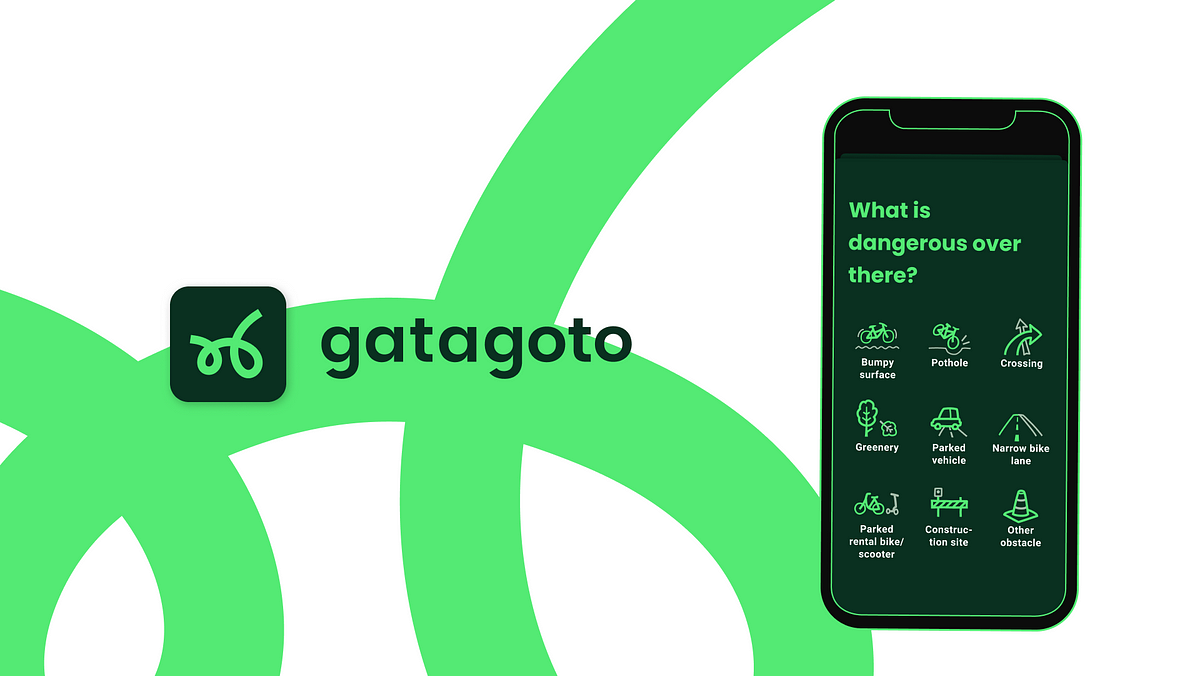
Technologies
- Machine Learning – image recognition based obstacle detection
- RxSwift
- Kotlin
- Spring Boot/Kotlin
- IaC – Terraform
- AWS
Screen Sample
Latest reports flyover feature